How AI Can Contribute To A Sustainable Energy Supply
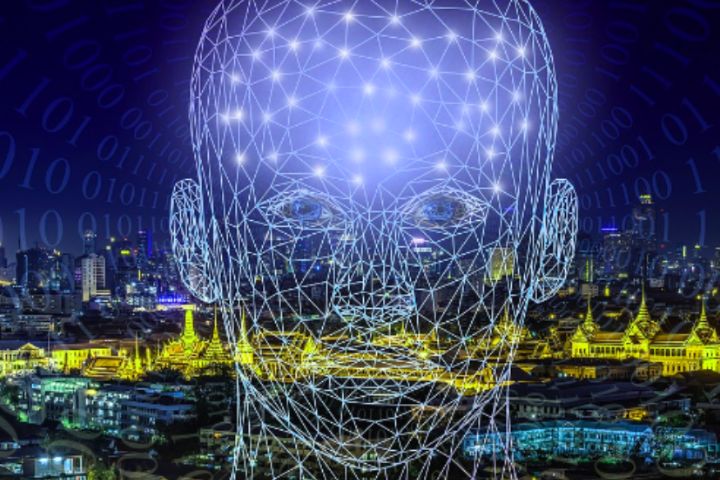
In combination with other technologies, artificial intelligence (AI) can contribute to a climate-friendly and efficient energy supply. With the change in our energy systems from large nuclear and coal-fired power plants to many small photovoltaic and wind power plants, distributed structures that require increasingly decentralized control are emerging. Another challenge when expanding renewable energy sources: The weather significantly impacts energy generation, so more excellent systems’ flexibility is needed. With the help of AI systems, we can meet the new requirements in our energy system.
Our energy systems are undergoing a change that is accompanied by increasing complexity. The coordination of energy generation and consumption can only be successful in the future if the designs are entirely digitized. The energy research department of the Oldenburg IT institute OFFIS is researching how digitization and AI systems can make our energy systems more efficient and resilient.
For example, AI machine learning methods can be used to evaluate weather data. Based on this data, forecasts for the feed-in of weather-dependent consumers can then be made. Such a forecast can then be used to adjust the generation and consumption of other assets to avoid curtailment and thus maximize the use of green electricity. In its white paper “Sustainable business models with artificial intelligence” and on its AI map, the platform learning systems show further practical examples of how safe and efficient management of power grids is made possible by AI-based predictions.
Table of Contents
New Challenges In The Sustainable Power Grid
While large power generation plants, such as nuclear and coal-fired, are connected to high and extra-high voltage grids, decentralized power generation from renewable energies occurs at lower voltage levels in the distribution grids. As a result, the distribution grids are currently being used in a way they were not designed for. There is little measurement and sensor technology on the lower network levels for monitoring the operating status. So far, the mathematical design of the operation was sufficient since the energy from the central power plants was routed to the consumers from top to bottom, and no feed-ins took place. Electric vehicles, for example, now pose a problem for the grids since their high consumption adds to the unpredictable feed-ins. In order to be able to recognize and prevent impending overloads of network elements in good time, the distribution networks’ status must be transparent. However, the vast expansion of sensors in the distribution grids is considered uneconomical. AI systems can make a significant contribution here. With neural networks based on the AI method of deep learning, the network states can be predicted more reliably and precisely, even if there is no comprehensive knowledge of the respective power supply.
Grid bottlenecks can also be avoided by intelligently coordinating the many tiny systems. An example of this can be found in electric vehicles, which increase the risk of overloading. These are often charged after work, so a lot of energy is required at once. In most cases, however, these vehicles can be set at different times, leading to network bottlenecks. Instead, in most cases, it is sufficient if the cars are fully charged the following day through intelligent coordination.
AI systems can provide further support in the area of data protection. For example, small intelligent systems, such as a homeowner’s heat pump, must not allow any conclusions to be drawn about sensitive information. In the homeowner’s case, for example, burglars could use the data to derive the vacation times of the resident to find a suitable time for the burglary. One solution is distributed self-organized systems in which each relevant unit in the power grid is equipped with AI software. This system can then collect the required data from the heat pump as an “agent”, interacting with other intelligent devices to solve problems and make autonomous decisions at a local level.
More Security And Stability Through Distributed AI
The battery storage swarm, which OFFIS developed in close cooperation with partners from the field, is an example of such a system. Battery storage systems are used at different locations, such as optimizing self-consumption in combination with photovoltaic or wind systems and for peak load shaving for industrial consumers. However, battery storage systems offer even more application possibilities. Each storage has been equipped with a digital agent that knows its battery storage’s characteristics and status for multi-purpose use. In addition, based on historical data and machine learning, he can create forecasts about the generation or consumption of customer systems on-site. The agent can then determine when and to what extent the battery storage is required for its primary application and whether it can be used for other purposes at other times of the day. In addition, the agents can self-organize their obligations among themselves if an agent cannot meet their commitment from the secondary market due to a forecast deviation.
There are several advantages to using the agent system, as this example shows. Only the necessary data is transmitted since the agents process the information locally. This ensures data protection, increases scalability and reduces the communication effort. In addition, these systems are particularly robust since the functionality of the entire system is not endangered by the failure of individual agents. Flexibility is also a strength. Self-organized systems can solve problems locally if unexpected changes occur. They thus contribute to stable network operation in the ongoing energy transition and represent a valuable component of our future digitized energy systems.
Current research also discusses the challenges associated with using AI in the energy system. A central question is how AI systems can be protected against attacks. In addition, decisions made by AI systems must be understandable for humans, significantly when critical infrastructure is affected. How the collective behavior of self-organized systems can be explained, justified and verified is, therefore, also the subject of research.
Also Read : Transfer Learning